AI in Battery Manufacturing: Faster Production of Critical Battery Materials
Increasing demand for electric vehicles, the expansion of renewable energy infrastructure, and the need for more efficient storage systems has catalyzed the manufacturing of critical battery materials. At present, most of these materials are produced overseas, and the US is heavily investing in promoting US sourced critical battery materials manufacture. One novel source of these materials is discarded EV batteries. However, diagnostics and testing to determine whether an EV battery is suitable for recycling can take from a few hours to a few days. By using AI, it is possible to optimize the manufacturing of critical battery materials by up to 25x through faster state of health (SoH) prediction (Najera-Flores et al. 2023).
Circular Supply Chains
Currently, the manufacturing industry follows a linear production model; raw materials are extracted and processed into products that get consumed. These products are then disposed of once they have served their purpose. Decarbonizing the US economy requires materials to be utilized beyond a single lifetime. Circular economy approaches can decrease the lifetime energy cost of materials and have a significant impact on global emissions (Ellen MacArthur Foundation 2019). Circular supply chains support regional manufacturing, enhance U.S. supply security, create jobs, and mitigate environmental impacts from landfilling, incineration, and extraction. However, these chains are complex due to varying regional factors and changing waste stream compositions over time. It is preferable to expand the lifespan of manufactured products, thereby retaining the inherent manufacturing value. When this is no longer possible, the aim is to recycle the products as quickly and efficiently as possible.
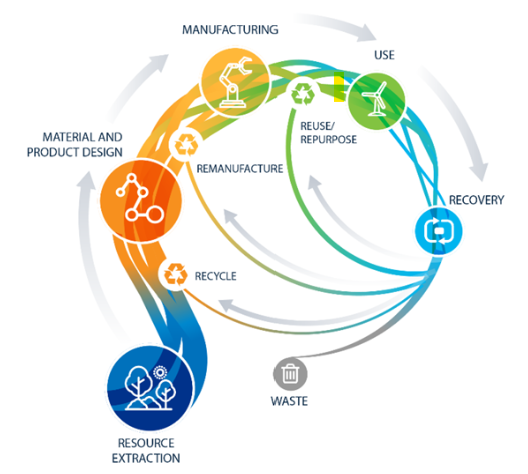
Taken from: Shubhankar Upasani, et al. 2022. Mapping the Opportunity Space to Model the Circular Economy Using Tools Funded by the DOE Office of Energy Efficiency and Renewable Energy. Golden, CO: National Renewable Energy Laboratory. NREL/TP-6A20-81693. https://www.nrel.gov/docs/fy22osti/81693.pdf.
Bottlenecks in End-of-Life Testing
One major bottleneck in the recycling of EV batteries is testing and diagnosis to determine the end-of-life (EOL) status. Traditional methods, involving multiple charge and discharge cycles, are not only time-consuming but also costly and resource-intensive. The energy demands of this process can further shorten the battery’s life, exacerbating costs and environmental impact.
Machine Learning Models for Battery Performance Insights
AI techniques, such as predictive analytics and data-driven models, can be used to improve battery diagnostics and forecasting. Machine learning involves using past battery performance data to determine the lifetime of a new battery under the same conditions. This perspective is predictive, as it uses algorithms that assess past data to learn and make accurate predictions of the future to establish the expected battery lifetime. Additionally, artificial neural networks, which operate in a similar way to human brains, are critical in identifying intricate patterns and dynamic structures needed to predict battery life.
Accelerating Battery Life Cycle Testing with AI
Artificial Intelligence has the potential to improve testing and diagnosis for battery end-of-life by orders of magnitude using several advanced techniques. AI can also automate data acquisition and processing, making it possible to more quickly analyze everything and make a decision. Advanced simulation algorithms can model the behavior of the battery at the software and state levels when exposed to different stresses. This greatly decreases the amount of conduct testing required.
This is particularly important to determine where a particular second life battery fits into the circular supply chain. It is a challenging task due to cross coupling and dependency between aging mechanisms. A recent study (Faraji-Niri et al. 2023) used electrochemical impedance spectroscopy (EIS) testing and a machine learning (ML) approach to predict SoH of Li-ion cells with an error of 1.1%. In another study (Takahashi, Allam, and Onori 2023), researchers proposed a machine learning-based approach for a quick prognosis of whether a retired electric vehicle’s battery is suitable for reuse or should be recycled. The study presents a novel algorithm that combined Gaussian process regression with bagging to analyze the battery data. This was validated with large datasets. This method provides extremely accurate predictions, with performance errors even for the most difficult cases amounting to no more than 1.48%.
The Future of AI in Battery Manufacturing
AI ecosystems represent another major step forward for the industry. The adoption of advanced machine learning models and neural networks now allows companies to forecast battery life, improve manufacturing processes, and enhance recycling efficiency to previously unattainable levels. As the production of electric vehicles and renewable energy solutions increases, AI becomes increasingly crucial for sustainable global development. AI spurs business and creativity and acts as a prerequisite for maintaining competitiveness in the global marketplace. This shift towards AI-augmented operations is not merely a trend but a seismic transformation that is reshaping battery manufacturing and associated sectors.
James White PhD and Joseph Moniodis PhD | 29th April 2024
Citation: James White and Joseph Moniodis. 2024. “AI In Battery Manufacturing: Faster Production Of Critical Battery Materials.” Carbon Critical. https://carbon-critical.com/ai-in-the-manufacturing-of-critical-battery-materials/
References
Ellen MacArthur Foundation. 2019. “Completing the picture: How the circular economy tackles climate change.” https://www.ellenmacarthurfoundation.org/completing-the-picture.
Faraji-Niri, Mona, Muhammad Rashid, Jonathan Sansom, Muhammad Sheikh, Dhammika Widanage, and James Marco. 2023. “Accelerated state of health estimation of second life lithium-ion batteries via electrochemical impedance spectroscopy tests and machine learning techniques.” Journal of Energy Storage 58. https://doi.org/10.1016/j.est.2022.106295.
Najera-Flores, David A., Zhen Hu, Mayank Chadra, and Michael D. Todd. 2023. “A Physics-Constrained Bayesian neural network for battery remaining useful life prediction.” Applied Mathematical Modelling 122:42-59. https://doi.org/10.1016/j.apm.2023.05.038.
Takahashi, Aki, Anirudh Allam, and Simona Onori. 2023. “Evaluating the feasibility of batteries for second-life applications using machine learning.” iScience 26 (4): 106547. https://doi.org/10.1016/j.isci.2023.106547.
Upasani, Shubhankar, Julien Walzberg, Dwarak Ravikumar, Alberta Carpenter, Garvin Heath, Ulises Gracida-Alvarez, Thathiana Benavides, et al. 2022. “Mapping the Opportunity Space to Model the Circular Economy Using Tools Funded by the DOE Office of Energy Efficiency and Renewable Energy,” NREL/TP-6A20-81693. National Renewable Energy Laboratory. https://www.nrel.gov/docs/fy22osti/81693.pdf.